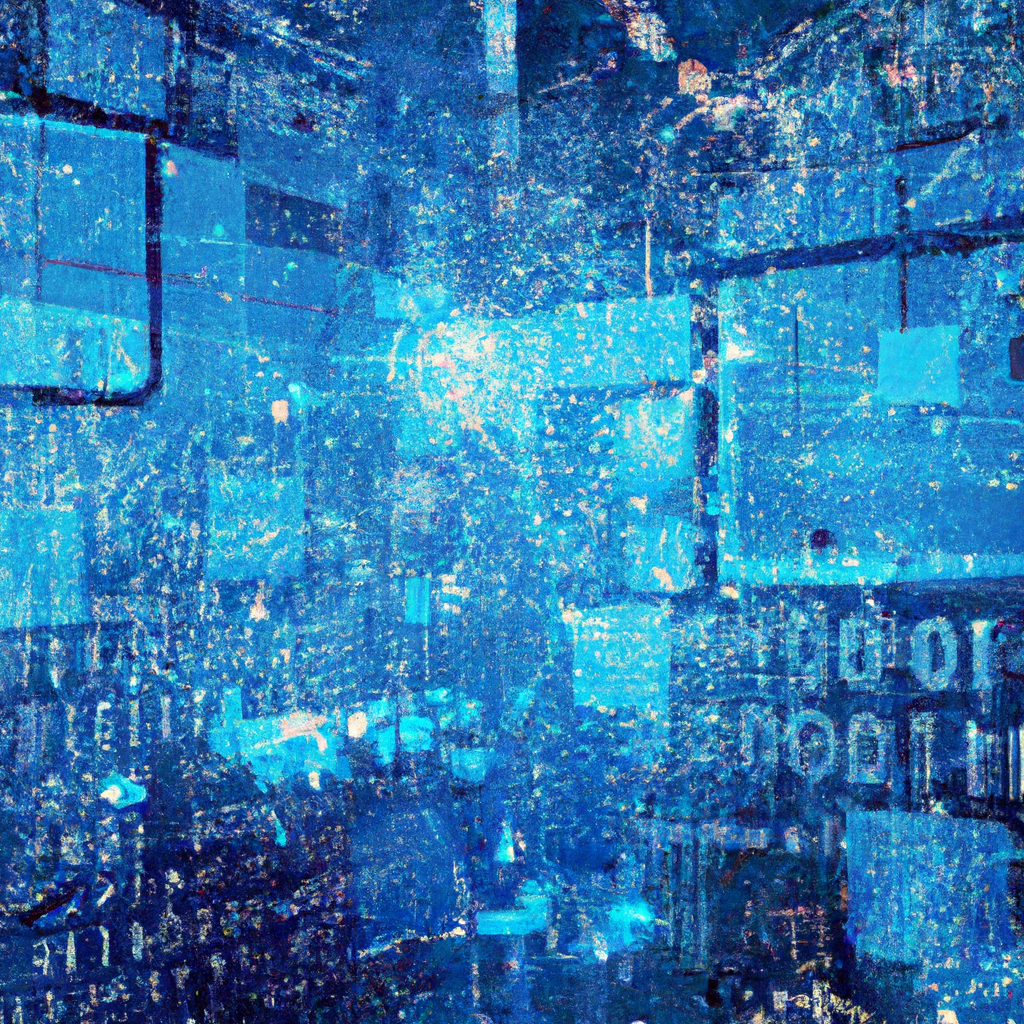
In today’s digital world, where fraud is becoming increasingly sophisticated, businesses need effective tools to detect and prevent fraudulent activities. Artificial Intelligence (AI) has emerged as a powerful tool in the fight against fraud. By leveraging AI algorithms and machine learning, businesses can now detect even the most intricate fraud schemes and safeguard their operations. This article explores how AI can be used to detect fraud, highlighting its benefits and providing practical tips on implementing AI-based fraud detection systems. Discover the revolutionary potential of AI in combating fraud and ensure the security of your business.
Understanding Fraud
Fraud is a deceptive or dishonest act, intentionally carried out to gain unauthorized benefits or deceive another person or entity. It can occur in various forms and industries, impacting individuals, companies, and the overall economy. It is crucial to have a comprehensive understanding of the common types of fraud and the importance of detecting and preventing it.
Common Types of Fraud
Fraud can manifest in different ways, but some common types include identity theft, credit card fraud, insurance fraud, and financial statement fraud. Identity theft involves stealing someone’s personal information to commit fraudulent activities, such as opening fraudulent accounts or making unauthorized purchases. Credit card fraud occurs when someone uses another person’s credit card details without their consent. Insurance fraud involves making false claims to receive insurance payouts. Financial statement fraud occurs when a company manipulates its financial records to present false information.
Importance of Detecting Fraud
Detecting fraud is paramount as it safeguards individuals and organizations from financial losses, reputational damage, and legal consequences. Fraudulent activities can have a devastating impact on victims’ lives, causing financial distress and emotional trauma. For companies, fraud can result in significant financial losses, damage to brand reputation, and loss of customer trust. Detecting fraud also helps maintain the integrity and transparency of financial systems, ensuring a fair playing field for all participants. By leveraging advanced technologies like Artificial Intelligence (AI), detecting and preventing fraud becomes more effective and efficient.
Introduction to AI in Fraud Detection
AI, or Artificial Intelligence, has revolutionized various industries, including fraud detection. By harnessing the power of AI, organizations can analyze vast amounts of data, identify patterns, and detect fraudulent activities with greater accuracy and speed. Whether in the financial sector, insurance industry, or e-commerce, AI brings tremendous advantages in the battle against fraud.
Advantages of AI in Fraud Detection
AI offers several advantages in fraud detection. Firstly, it enables real-time monitoring of transactions and activities, allowing prompt identification of suspicious behavior. Secondly, AI algorithms can analyze large datasets that humans might find overwhelming, identifying intricate patterns and anomalies that signify fraud. The speed and efficiency of AI in processing vast amounts of data greatly enhance fraud detection capabilities. Additionally, AI can continuously learn and adapt, improving its accuracy over time. This adaptability enables AI systems to stay updated with emerging fraud techniques and stay one step ahead of criminals.
How AI Works in Fraud Detection
AI systems for fraud detection utilize various techniques such as supervised machine learning, unsupervised learning, deep learning, natural language processing (NLP), and predictive analytics. These techniques enable AI systems to analyze data, identify fraudulent patterns, and make accurate predictions.
Data Collection and Preparation
One crucial aspect of AI in fraud detection is the collection and preparation of relevant data. Gathering accurate and comprehensive data sets the foundation for developing effective fraud detection models.
Gathering Relevant Data
To train an AI model for fraud detection, organizations need to collect data that represents both genuine and fraudulent transactions. This data should include various attributes related to the transactions, such as customer information, transaction amount, location, and time. By having a diverse range of data, AI models can learn to identify patterns and behaviors that indicate potential fraud.
Data Cleaning and Validation
Once the data is gathered, it is essential to clean and validate the data. This process involves removing any duplicate or erroneous entries and ensuring data integrity. Clean and validated data is crucial for accurate model training, as it minimizes the influence of incorrect or misleading information.
Supervised Machine Learning in Fraud Detection
Supervised machine learning plays a crucial role in training AI models for fraud detection. It involves feeding the AI system labeled data, where each transaction or activity is tagged as genuine or fraudulent.
Training Data Sets
Creating training data sets involves labeling a significant number of transactions as genuine or fraudulent. Organizations can leverage historical transaction data, past fraud cases, or expert knowledge to label the data accurately. The larger and more diverse the training data set, the better the AI model’s ability to identify fraudulent patterns accurately.
Selection of Algorithms
Various machine learning algorithms can be applied to train AI models for fraud detection. These algorithms include logistic regression, decision trees, random forests, and support vector machines (SVM). Each algorithm has its strengths and weaknesses, making it essential to select the most suitable ones based on the organization’s specific needs and data characteristics.
The Role of Unsupervised Learning
Unsupervised learning complements supervised machine learning techniques in fraud detection. Unlike supervised learning, unsupervised learning does not require labeled data and can identify patterns and anomalies in data independently.
Applying Clustering Techniques
Clustering techniques, such as k-means clustering and hierarchical clustering, help identify groups or clusters of similar transactions. These clusters can reveal patterns that indicate potential fraudulent behavior. By analyzing the characteristics shared by transactions in a cluster, AI models can identify suspicious activity that deviates from the norm.
Outlier Detection
Outlier detection techniques aim to identify individual transactions or activities that significantly differ from the majority of data points. These outliers often represent unique or abnormal behavior, which could indicate fraudulent activities. AI systems can use algorithms like Isolation Forest or Local Outlier Factor to detect and flag such outliers for further investigation.
Deep Learning for Fraud Detection
Deep learning, a subfield of AI, has gained significant popularity and success in various domains, including fraud detection. Deep learning models, such as neural networks and convolutional neural networks (CNN), have the ability to process vast amounts of data and identify complex patterns.
Neural Networks
Neural networks are composed of interconnected layers of artificial neurons, inspired by the human brain’s structure. They can capture intricate relationships and nonlinear patterns in data. In fraud detection, neural networks can analyze transaction attributes and detect fraud based on learned patterns and anomalies.
Convolutional Neural Networks
Convolutional Neural Networks (CNNs) are highly effective in processing structured data, such as images and sequences. In fraud detection, CNNs can analyze patterns within transaction data, identifying potential fraudulent behavior based on abnormal sequences or attributes.
Natural Language Processing in Fraud Detection
Natural Language Processing (NLP) empowers AI systems to understand and analyze human language, enabling them to extract meaning from textual data related to fraud detection.
Sentiment Analysis
Sentiment analysis allows AI systems to determine the sentiment or emotional tone behind text, such as customer reviews or social media posts. By analyzing sentiment, AI models can identify potential fraudulent activities, such as fake reviews or misleading promotional content.
Text Classification
Text classification techniques help categorize textual data into different classes or categories. In the context of fraud detection, text classification can help identify text-based fraud, such as phishing emails or fraudulent advertisements. By automating the classification process, AI systems can streamline fraud detection efforts.
Utilizing Predictive Analytics
Predictive analytics leverages historical data and statistical modeling techniques to make predictions about future events. In fraud detection, predictive analytics helps identify potential fraudulent activities based on patterns and trends.
Regression Techniques
Regression techniques allow organizations to analyze the relationship between dependent and independent variables, enabling predictions of future outcomes. In fraud detection, regression models can analyze historical transaction data and identify patterns that suggest potential fraudulent behavior.
Time Series Analysis
Time series analysis focuses on analyzing data points collected over time to identify patterns, trends, and seasonality. In fraud detection, time series analysis can help detect anomalies or sudden changes in transaction patterns that may indicate fraudulent activities.
Real-time Fraud Detection with AI
Detecting fraud in real-time is often crucial to prevent financial losses and mitigate risks. AI systems can enable real-time fraud detection through stream processing and rule-based systems.
Stream Processing
Stream processing involves analyzing and processing data in real-time as it is generated. By applying AI algorithms to the incoming stream of data, organizations can detect and flag potentially fraudulent transactions as they occur. Stream processing enables immediate action and intervention to prevent further damage.
Rule-based Systems
Rule-based systems in fraud detection utilize predefined rules or patterns to identify potential fraud. These rules are based on expert knowledge and industry best practices. AI systems can rapidly apply these rules to incoming data and flag transactions that match the predefined patterns. Rule-based systems provide a quick and configurable way to detect fraud in real-time.
Challenges and Limitations of AI in Fraud Detection
Although AI brings tremendous advantages to fraud detection, it also faces several challenges and limitations that warrant attention.
Data Privacy and Protection
Handling sensitive and personal data in fraud detection requires strict adherence to data privacy laws and regulations. Organizations must ensure that data collection, storage, and analysis processes comply with applicable standards to protect individuals’ privacy.
Adaptability to New Fraud Techniques
As fraudsters continuously develop new techniques and strategies, AI systems must adapt and evolve to detect emerging fraud patterns effectively. Regular updates and ongoing monitoring are crucial to ensure AI systems remain effective in combating new fraud methods.
In conclusion, AI has proven to be a game-changer in fraud detection. It enables the analysis of vast amounts of data, identification of patterns, and detection of anomalies that signal potential fraud. With techniques such as supervised machine learning, unsupervised learning, deep learning, natural language processing, and predictive analytics, AI systems can significantly enhance fraud detection capabilities. However, it is important to address challenges like data privacy and system adaptability to maximize the efficacy of AI in combating fraud. By harnessing the power of AI, organizations can stay one step ahead of fraudsters, protecting themselves and their customers from financial losses and reputational damage.