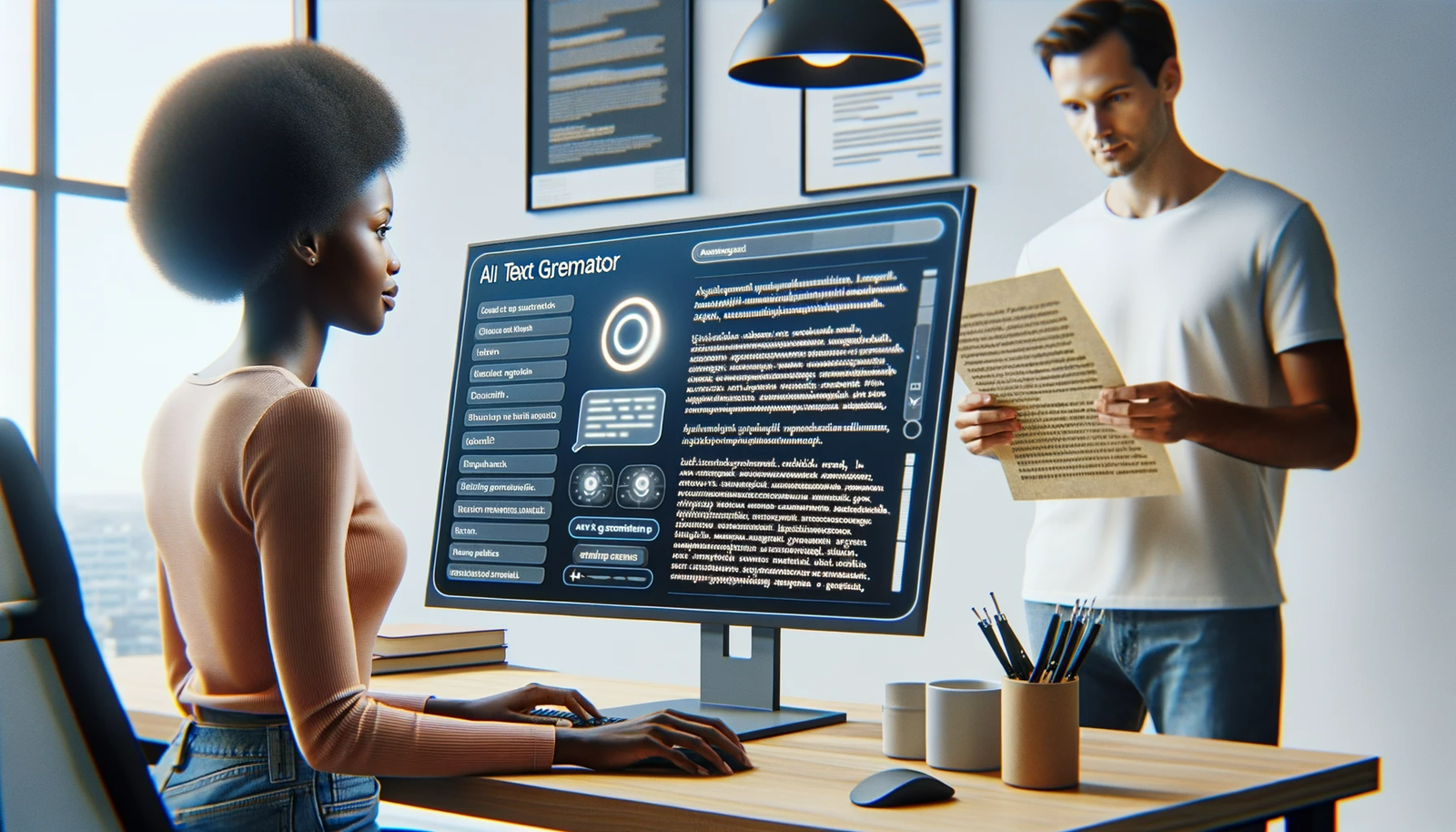
In today’s digital age, AI technology has revolutionized various industries and opened up new possibilities. One such advancement is the ability to use AI to generate text. If you’ve ever wondered how you can leverage AI to effortlessly create captivating written content, you’re in for a treat. This article will guide you through the fascinating world of using AI to generate text, providing you with valuable insights and techniques to enhance your writing endeavors. Get ready to unlock the potential of AI and take your writing to the next level!

Understanding AI Text Generation
AI text generation refers to the process of using artificial intelligence algorithms and models to generate human-like text. These algorithms are designed to analyze and understand patterns, structures, and relationships in existing text data, and then generate new text that follows similar patterns. This technology has gained significant attention in recent years for its potential in various fields, including content creation, customer service, and language translation.
Different methods of AI text generation
There are several methods that can be utilized for AI text generation. One popular approach is using recurrent neural networks (RNNs), which are designed to process sequential data such as text. RNNs can learn from large datasets and generate text based on the patterns and structure they discover. Another method is the use of transformer models, such as OpenAI’s GPT (Generative Pre-trained Transformer). These models, powered by deep learning algorithms, are capable of generating highly coherent and contextually accurate text.
Applications of AI text generation
AI text generation has a wide range of applications in different industries. One of the most prominent applications is content creation. With the ability to generate coherent and contextually relevant text, AI can assist in producing articles, blog posts, product descriptions, and social media content. This can help businesses save time and effort on content creation while still maintaining quality and relevance.
AI text generation also has potential in customer service. Chatbots and virtual assistants can be trained to generate natural language responses to customer inquiries, providing an efficient and personalized customer experience. Additionally, AI text generation can be used for language translation, where it can generate accurate translations by learning from large multilingual datasets.
Preparing Data for AI Text Generation
Before training an AI text-generation model, it is crucial to prepare the data. Collecting and cleaning data involves gathering a diverse range of text samples that are relevant to the desired text generation task. This can include books, articles, or any other source that provides a broad representation of the text required. Cleaning the data involves removing any unnecessary noise or irrelevant information that may affect the model’s training.
Deciding on the scope and style of the generated text is also essential. This involves determining the type of text the model will generate, whether it is creative writing, technical documentation, or poetry. Furthermore, formatting the data for machine learning models involves transforming the text into a suitable format that can be processed and used by the AI model during training.
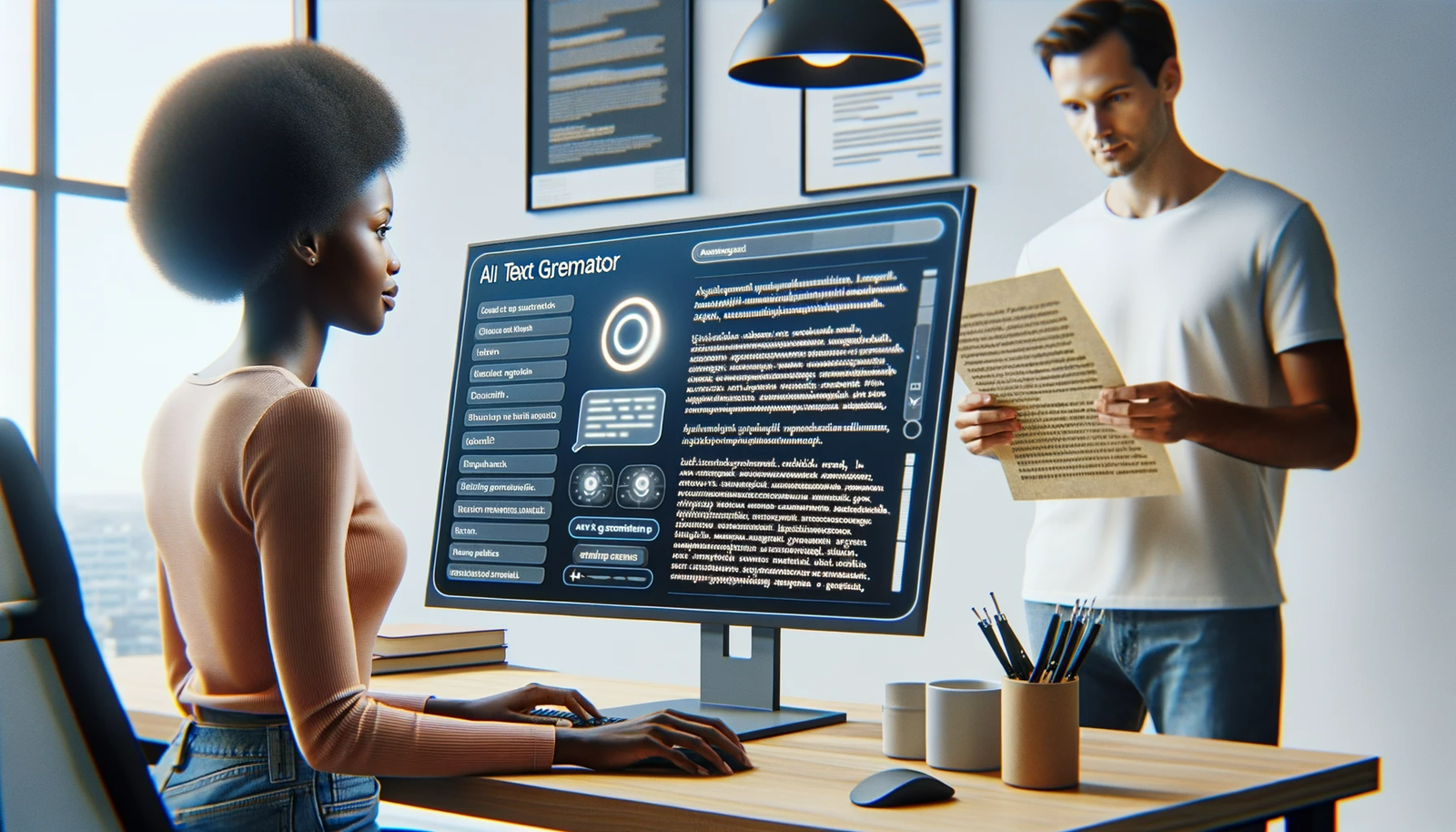
Choosing the Right AI Text Generation Model
Choosing the right AI text generation model depends on the specific requirements of the task. Exploring popular models such as GPT-3, GPT-2, and BERT can provide insights into their capabilities and limitations. Comparing the strengths and weaknesses of these models can help determine the model that best aligns with the desired outcomes.
Evaluating model performance involves assessing various metrics such as fluency, coherence, and relevance. This can be done using human evaluators or automated evaluation metrics. By evaluating model performance, it is possible to refine and select the most suitable AI text generation model.
Training an AI Text Generation Model
Training an AI text generation model requires selecting a suitable training dataset. The dataset should include diverse and representative samples of the type of text the model is expected to generate. It is essential to balance the dataset to avoid biases and ensure fairness in the generated text.
Configuring hyperparameters is another crucial step in training an AI text generation model. Hyperparameters control the behavior of the model during training, such as the learning rate, batch size, and the number of training iterations. Experimenting with different hyperparameters can help fine-tune the model’s performance.
Creating a training pipeline involves preprocessing the data, splitting it into train and validation sets, and feeding it into the model for training. This process requires optimizing computational resources and monitoring the model’s progress to ensure it is learning effectively.
Fine-tuning AI Text Generation Models
Fine-tuning an AI text generation model involves adapting a pretrained model to specific text generation tasks. This approach is useful when the dataset available for training is limited or when the task requires generating text with a specific context or style.
Understanding the concept of fine-tuning is important for selecting the right pretrained model. Pretrained models such as GPT-3 or BERT can be fine-tuned by using domain-specific data or by including additional training steps on task-specific data.
Adapting the model to specific text generation tasks can involve providing task-specific prompts or instructions to guide the generation process. This allows for control and customization of the generated text while ensuring it aligns with the desired objectives.
Generating Text with AI Models
Generating text with AI models involves setting up the input and output formats. Input formats vary depending on the model and can include plain text or structured data. Output formats can be specified to meet specific requirements, such as generating text in a certain language or format.
Using prompts and instructions can guide the model to generate text with specific outputs. By providing clear instructions and context, it is possible to steer the model’s generated text in a desired direction. This can be useful for tasks such as writing summaries, generating responses, or creating content within a specific theme.
Controlling randomness and creativity in generated text can be achieved through techniques such as temperature adjustment. Higher temperatures result in more random and creative outputs, while lower temperatures yield more focused and deterministic results. Balancing these adjustments allows for fine-tuning the model’s output to meet individual preferences.
Evaluating and Refining AI Text Generation
Evaluating the quality and coherence of generated text is crucial to ensure it meets the desired standards. Assessing metrics like fluency, coherence, and relevance can be done through manual evaluation by human experts or through the use of automated evaluation metrics.
Iterating and improving the model is an ongoing process that involves analyzing feedback and making necessary adjustments. User feedback plays a vital role in identifying areas for improvement and fine-tuning the model accordingly. By continuously learning and refining the model, it can generate even more accurate and contextually appropriate text.
Incorporating user feedback into the training process can further enhance the performance of the AI text generation model. This feedback loop allows for continuous improvement and adaptation to user preferences and requirements.
Ethical Considerations in AI Text Generation
Addressing biases and discrimination in generated text is a critical ethical consideration. AI models are trained on data, and if this data contains biases or discriminatory content, the model may inadvertently generate text that perpetuates those biases. It is important to carefully curate and balance the training data to ensure fairness, inclusivity, and avoid reinforcing negative stereotypes.
Avoiding unethical uses of AI-generated content is essential. AI text generation should not be employed to deceive, manipulate, or spread false information. Ensuring the responsible use of the technology is crucial in maintaining trust and integrity.
Establishing transparency and disclosure practices is vital in AI text generation. Users interacting with AI-generated content should be aware that they are engaging with an AI system and not a human. Transparency helps build trust and mitigates the risk of creating false expectations or confusion.
Limitations and Challenges in AI Text Generation
Understanding the limitations of AI text generation is essential for managing expectations. Generating long-form content can be challenging for AI models, as they may struggle with maintaining coherence and consistency over extended lengths. Therefore, it is important to consider the length and complexity of the desired output when using AI text generation.
Handling context and generating accurate information are additional challenges. AI models may generate text that is contextually incorrect or provides inaccurate information. Addressing these challenges requires careful training and fine-tuning to ensure the model understands and captures the nuances of the context.
Overcoming biases and lack of diversity in training data is crucial for fair and inclusive AI text generation. Biases in training data, whether based on gender, race, or other factors, can lead to biased text generation. It is important to actively address these biases by collecting diverse and representative datasets, as well as using bias correction techniques during training.
Best Practices for AI Text Generation
Ensuring legal and copyright compliance is essential when using AI text generation. It is important to respect intellectual property rights and avoid plagiarism. Properly attributing and obtaining permissions when necessary helps maintain ethical standards and legal compliance.
Using AI-generated text as a starting point for human refinement can enhance the quality and coherence of the output. Human editors and reviewers can review, edit, and improve the generated text to ensure it meets higher standards of quality and relevance.
Continuously learning and adapting with new techniques is key to staying at the forefront of AI text generation. The field of AI evolves rapidly, and new models and approaches are constantly emerging. Keeping up with the latest developments and incorporating them into the text generation process can lead to improved results and efficiency.
In conclusion, AI text generation holds immense potential in various industries and applications. Understanding the different methods, preparing the data, choosing the right model, training and fine-tuning, generating text, evaluating and refining, considering ethical implications and limitations, and following best practices are crucial steps to success in AI text generation. By harnessing the power of AI, we can unlock new levels of efficiency and creativity in generating human-like text.