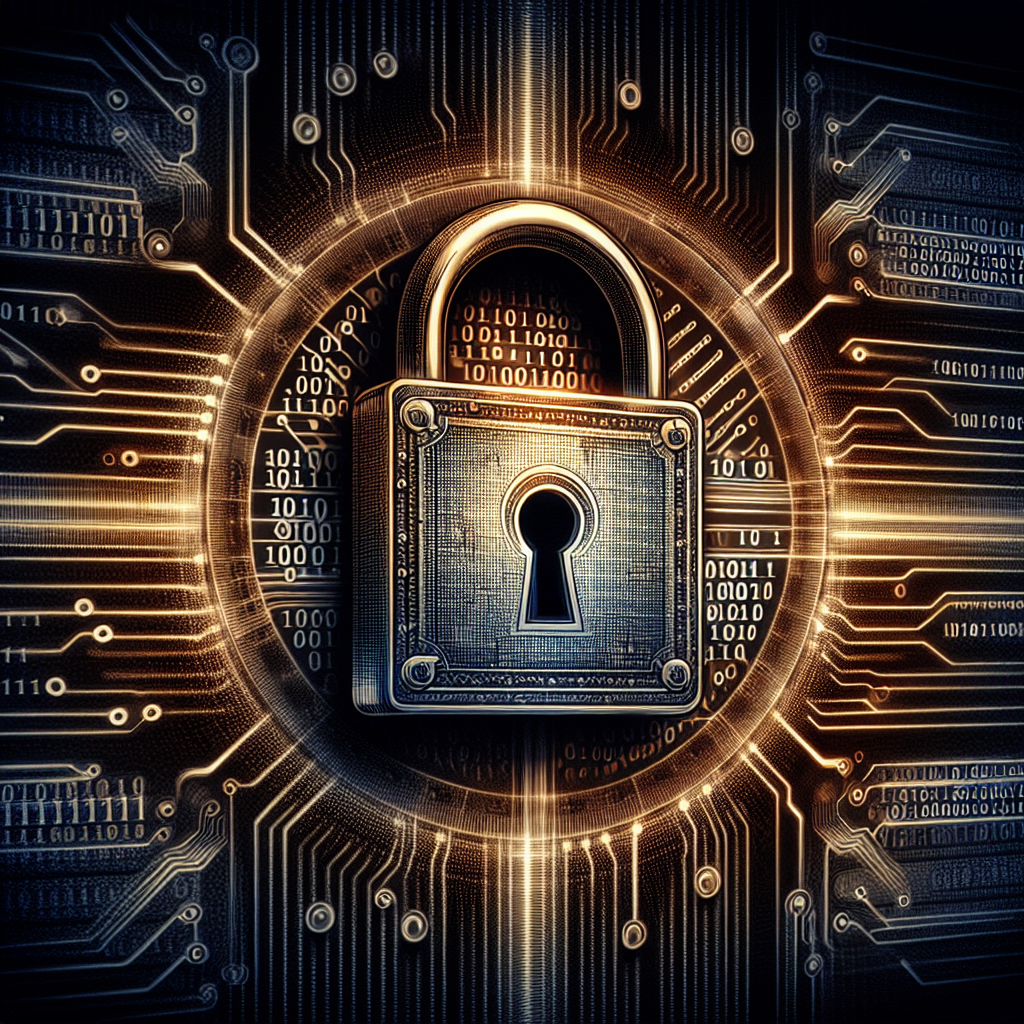
In today’s digital age, where artificial intelligence (AI) plays an increasingly prominent role, the issue of data privacy has become a pressing concern. The impact of data privacy on AI applications is a topic gaining traction, as individuals and organizations alike grapple with how to navigate the delicate balance between accessing and protecting personal information. This article explores the concerns surrounding data privacy in AI applications and provides potential solutions to safeguard sensitive data while harnessing the power of AI. Data Privacy and AI: Concerns and Solutions Regarding Data Privacy in AI Applications
With the rapid advancement of artificial intelligence (AI) technologies, data privacy has become a significant concern when it comes to AI applications. AI relies heavily on data, and as such, there are numerous concerns related to the privacy and security of personal information. In this article, we will explore the concerns, ethical implications, legal frameworks, and data protection measures surrounding data privacy in AI applications. Additionally, we will discuss the impact of data privacy on AI training and development, ethical considerations in AI applications, data privacy in AI-enabled services, emerging technologies and data privacy, data privacy in AI research, risks and challenges in data privacy for AI applications, benefits of data privacy for AI applications, collaborative solutions and partnerships, as well as the future of data privacy in AI applications.
Concerns Related to Data Privacy in AI Applications
Data privacy concerns in AI applications are primarily centered around the collection, storage, and usage of personal data. As AI algorithms increasingly rely on large datasets for training, there is a risk of unauthorized access to sensitive information. This raises concerns about potential data breaches, identity theft, and misuse of personal data. Additionally, the lack of transparency in AI systems makes it difficult for individuals to understand how their data is being used, leading to privacy concerns.
Ethical Implications of Data Privacy in AI Applications
The ethical implications of data privacy in AI applications are closely tied to concerns about bias and discrimination. AI algorithms can unwittingly perpetuate bias if trained on biased datasets, resulting in unfair and discriminatory outcomes. Ensuring the protection of sensitive information is also an ethical consideration, as the unauthorized disclosure of such data can have severe consequences for individuals. Furthermore, informed consent and user control over personal data are important ethical considerations in AI applications.
Legal Frameworks for Data Privacy in AI Applications
In response to the increasing concerns surrounding data privacy in AI applications, many countries and regions have implemented or are in the process of implementing legal frameworks to regulate the collection, storage, and usage of personal data. For example, the European Union’s General Data Protection Regulation (GDPR) sets out strict guidelines for the processing of personal data, including AI applications. These regulations aim to protect individuals’ rights and provide transparency and accountability in the use of personal data.
Data Protection Measures in AI Applications
To address the concerns related to data privacy in AI applications, various data protection measures can be implemented. One such measure is data anonymization, which involves removing or encrypting personally identifiable information from datasets used for AI training. This helps protect individuals’ privacy while still allowing for the utilization of data in AI development. Another important measure is data minimization, which involves only collecting and retaining the necessary data for a specific purpose. Additionally, robust cybersecurity measures and access controls can help prevent unauthorized access to personal data in AI applications.
Impact on AI Training and Development
The impact of data privacy on AI training and development is substantial. Availability of diverse and representative data is crucial for training AI algorithms to produce unbiased and accurate results. However, concerns about privacy may limit the availability of certain types of data, particularly when it comes to sensitive information. Moreover, data privacy regulations and compliance challenges can impose limitations on the access and usage of data for AI purposes.
Availability of Diverse and Representative Data
To ensure the accuracy and fairness of AI algorithms, it is essential to have diverse and representative datasets during the training process. However, data privacy concerns may restrict access to certain types of data, leading to biased AI systems. Addressing this challenge requires striking a balance between data privacy and the need for inclusive and representative datasets.
Impact on AI Algorithms and Models
Data privacy considerations can have a significant impact on AI algorithms and models. The exclusion of certain sensitive data may lead to gaps in the learning process, potentially affecting the performance and accuracy of AI systems. This raises the need for innovative techniques that can preserve data privacy while still enabling effective AI training and development.
Data Privacy Regulations and Compliance Challenges
Data privacy regulations, such as the GDPR, impose certain obligations on organizations using AI systems. Compliance with these regulations can pose challenges, especially for organizations operating in multiple jurisdictions with different privacy laws. Adhering to these regulations without compromising the functionality and utility of AI systems requires careful management of personal data and robust privacy practices.
Ethical Considerations in AI Applications
In addition to data privacy concerns, ethical considerations play a crucial role in AI applications. Two critical ethical considerations are the potential for bias and discrimination in AI systems and ensuring informed consent and user control over personal data.
Bias and Discrimination
AI systems that are trained on biased datasets can perpetuate and amplify societal biases, leading to discriminatory outcomes. To address this, it is important to ensure that AI algorithms are developed using diverse and representative datasets and regularly audited for biases. Moreover, ongoing monitoring and evaluation of AI systems in real-world scenarios can help identify and rectify any biases that emerge.
Informed Consent and User Control
Respecting individuals’ autonomy and giving them control over their personal data is essential in AI applications. Transparent information about data collection, usage, and potential risks should be provided to users, enabling them to make informed decisions about whether to share their data or not. User-friendly mechanisms for controlling and managing personal data should be implemented, empowering individuals to exercise their rights and maintain control over their privacy.
Protection of Sensitive Information
Many AI applications involve processing sensitive personal information, such as health or financial data. Protecting this sensitive information from unauthorized access and disclosure is crucial. Implementing robust security measures, including encryption and access controls, can safeguard sensitive data while still enabling AI development and usage.
Data Privacy in AI-enabled Services
AI-enabled services, such as virtual assistants, smart homes, and healthcare applications, rely on extensive data collection and analysis. Ensuring data privacy in these services is vital to maintain consumer trust and protect individuals’ personal information.
Virtual Assistants and Personalization
Virtual assistants, like voice-controlled AI devices, utilize personal data to provide personalized experiences and perform tasks on behalf of users. However, concerns about the collection and storage of sensitive data, as well as potential breaches of privacy, have raised questions about the balance between personalization and data privacy. Implementing strong security measures and giving users control over their data can help alleviate these concerns.
Smart Homes and Internet of Things (IoT)
Smart homes, equipped with IoT devices, gather a wealth of personal data to automate various functions, such as thermostats and security systems. Protecting this data from unauthorized access is essential to ensure privacy and prevent potential misuse. Secure data transmission, encryption, and regular software updates are some measures that can be implemented to protect data privacy in smart homes.
Healthcare and Medical Applications
In healthcare and medical applications, AI has the potential to revolutionize diagnostics, treatment, and patient care. However, the use of sensitive health information in AI systems raises concerns about privacy and confidentiality. Strict adherence to data protection regulations, such as HIPAA in the United States, along with robust security measures, is necessary to uphold patient privacy and trust.
Emerging Technologies and Data Privacy
As new technologies emerge, the intersection of data privacy and AI becomes even more complex. Three emerging technologies that have implications for data privacy in AI applications are blockchain and distributed ledger technology, federated learning, and homomorphic encryption.
Blockchain and Distributed Ledger Technology
Blockchain and distributed ledger technology offer potential solutions for enhancing data privacy in AI applications. By providing transparent and tamper-evident records of data transactions, blockchain can ensure data integrity and minimize the risk of unauthorized access or manipulation. Additionally, distributed ledger technology allows for decentralized storage and control of data, reducing the reliance on central authorities.
Federated Learning
Federated learning enables AI models to be trained on decentralized data sources without the need for data to be transferred to a centralized server. This approach can help address data privacy concerns by allowing individuals to maintain control over their data while still contributing to AI model training. By keeping the data local, federated learning preserves privacy while enabling collaborative AI development.
Homomorphic Encryption
Homomorphic encryption is a cryptographic technique that allows computations to be performed on encrypted data without decrypting it. This technology has the potential to enable privacy-preserving AI applications by ensuring that personal data remains encrypted throughout the processing pipeline. By eliminating the need to decrypt data, homomorphic encryption protects privacy while still allowing for AI analysis and insights.
Data Privacy and AI Research
Data privacy considerations in AI research are crucial for responsible data collection and use, as well as ensuring transparency and explainability.
Responsible Data Collection and Use
From the very beginning of AI research and development, responsible data collection practices should be followed. This involves obtaining informed consent from individuals, anonymizing data, and minimizing the collection of unnecessary personal information. Additionally, ensuring that AI systems are trained on diverse and representative datasets is vital to avoid biases and discriminatory outcomes.
Transparency and Explainability
AI systems should be transparent and explainable to users, allowing them to understand how decisions are made and how their personal data is being used. Explainability is essential to build trust and enable individuals to hold AI systems accountable. Providing clear and understandable explanations about the functioning of AI algorithms and the factors influencing decisions can help address concerns related to the opacity of AI systems.
Risks and Challenges in Data Privacy for AI Applications
Several risks and challenges arise when it comes to data privacy in AI applications, including data breaches and unauthorized access, lack of awareness and education, and international data transfers.
Data Breaches and Unauthorized Access
Data breaches pose a significant risk to data privacy in AI applications. If personal data is not properly protected, it can be subject to unauthorized access, theft, or manipulation. Robust cybersecurity measures, encryption, and access controls are crucial to minimize the risk of data breaches and protect individuals’ personal information.
Lack of Awareness and Education
Lack of awareness and education about data privacy among users and organizations can undermine the protection of personal information. Many individuals are unaware of the potential risks and implications of sharing their data in AI applications. Similarly, organizations may lack the necessary knowledge and resources to implement effective data privacy practices. Raising awareness and providing educational resources can help address this challenge.
International Data Transfers
As AI applications operate globally, international data transfers are common. However, data privacy regulations may differ between jurisdictions, raising challenges for organizations aiming to comply with multiple sets of laws. Mechanisms such as standard contractual clauses and binding corporate rules can facilitate lawful data transfers while ensuring the protection of personal data across borders.
Benefits of Data Privacy for AI Applications
While data privacy considerations bring about challenges, they also offer numerous benefits for AI applications. These benefits include enhanced user trust and satisfaction, improved accuracy and performance, and compliance with regulations.
Enhanced User Trust and Satisfaction
Data privacy measures, such as transparent data collection practices and user control over personal information, can enhance user trust and satisfaction. When individuals believe that their privacy is being respected and their personal data is being used responsibly, they are more likely to engage with AI applications and provide the necessary data for further development.
Improved Accuracy and Performance
Data privacy considerations can lead to the availability of diverse and representative datasets, which, in turn, can improve the accuracy and performance of AI systems. By training AI algorithms on datasets that encompass a wide range of demographics, biases can be minimized, leading to more fair and accurate outcomes.
Compliance with Regulations
Implementing data privacy measures ensures compliance with applicable regulations, such as the GDPR. By adhering to these regulations, organizations not only protect individuals’ privacy but also avoid legal consequences and reputational damage.
Collaborative Solutions and Partnerships
Addressing data privacy concerns in AI applications requires collaboration among various stakeholders, including government and regulatory agencies, industry alliances, and the development of privacy-enhancing technologies and tools.
Government and Regulatory Agencies
Government and regulatory agencies play a crucial role in addressing data privacy concerns through the development and enforcement of laws and regulations. Collaborative efforts between these agencies and the private sector can help create a comprehensive framework that protects individuals’ privacy while promoting innovation and AI development.
Industry Alliances and Standards
Industry alliances and standards organizations have an opportunity to work together to establish best practices and guidelines for data privacy in AI applications. By sharing knowledge and resources, these alliances can ensure that AI systems are developed and deployed with privacy safeguards in place.
Privacy-enhancing Technologies and Tools
The development and adoption of privacy-enhancing technologies and tools can significantly contribute to data privacy in AI applications. Techniques such as differential privacy, secure multi-party computation, and privacy-preserving machine learning can enable organizations to extract insights and train AI models while respecting individuals’ privacy.
The Future of Data Privacy in AI Applications
As AI technologies continue to evolve, the future of data privacy in AI applications will be shaped by the need to strike a balance between privacy and progress.
Striking the Balance between Privacy and Progress
As AI technologies advance, balancing privacy concerns with the potential benefits of AI applications will become increasingly important. Striking the right balance will require ongoing discussions and collaborations among stakeholders to ensure that privacy is protected while enabling the development and deployment of AI systems that can positively impact society.
Evolution of Data Privacy Regulations
Data privacy regulations are likely to evolve in response to the rapid advancement of AI technologies. Governments and regulatory agencies will continue to refine existing regulations and introduce new ones to keep pace with the changing landscape of AI and address emerging privacy challenges.
Advancements in Privacy-preserving AI Technologies
Advancements in privacy-preserving AI technologies will play a pivotal role in addressing data privacy concerns in AI applications. Continued research and development in areas such as federated learning, homomorphic encryption, and secure data sharing will enable organizations to leverage AI while protecting individuals’ privacy.
In conclusion, data privacy is a crucial consideration for AI applications. Concerns related to data privacy, such as unauthorized access and bias, ethical implications, and compliance with regulations, need to be addressed through the implementation of robust data protection measures. Balancing privacy and progress, evolving regulations, and advancements in privacy-preserving AI technologies will shape the future of data privacy in AI applications. By prioritizing data privacy, we can ensure the responsible and ethical development and usage of AI systems while safeguarding individuals’ personal information.